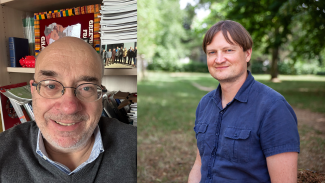
Today's Nobel Prize in Physics was awarded to John Hopfield and Geoffrey Hinton for their fundamental discoveries and contributions in the field of machine learning. Machine learning (ML) is a branch of artificial intelligence (AI) in which computers are used to learn from data and recognize patterns. ML algorithms use mathematical models to make predictions or decisions.
Can you explain today's Nobel Prize in the field of machine learning and AI research? To what extent is it justified?
Prof. Tkatchenko: “Machine Learning is a set of methods which is trained on data. It is trying to make a representation of the problem you are interested in, given data. That is very different from the traditional physical approach, where you first have a model with a fixed representation that you can then adjust with the data. Here, you do it the other way round. That change in perspective is quite revolutionary.
The first artificial neural networks that have been developed, the Hopfield networks (Ed. note: named after the current Nobel Prize winner, see infobox), came from statistical physics; they were the first generation. Then the algorithms were trained and made more powerful, and now they are used in all domains of science and technology. For that reason, for that fundamental, foundational development, the Nobel Prize is very justified.”
Prof. Schommer: “The Nobel Prize has been awarded to two scientists who have done impressive pioneering work. The first beginnings can be traced back to the 1960s. The basic idea was to use mathematical models to simulate neuronal activity, i.e. to imitate the functioning of the human brain. ML is not just a mathematical model, but an interdisciplinary discipline that is close to bionics: nature is taken as a template. It has developed fantastically over time.”
What influence did this technology have on your own career?
Prof. Tkatchenko: “My bachelor’s degree was in computer science, but neural networks at the time were not very impressive. They were already very interesting though as a tool for developers. You could use neural networks to compress songs in WAV format; that was before we had MP3. You lost some accuracy, but the song was recognisable.
10 years later, ML was a completely different field. It was not so much a single breakthrough, rather than multiple ones in many directions that revolutionised ML: computer hardware, software, algorithms… This is why NVIDIA is now a 3 trillion Dollar company! The computer architecture is becoming more and more powerful, and that makes our progress in quantum chemistry and physics possible.”
Prof. Schommer: “The 1980s were characterized by the development of AI in general and neural networks in particular. At that time, I studied Hopfield networks (Ed. Note: named after the current Nobel Prize winner, see infobox), other neural network architectures and connectionism. I was immediately fascinated by the question of whether and if so, how we could artificially simulate cognitive problem solving. In one of my papers, I dealt with the disambiguation of multiple-meaning sentences, such as syntactically ambiguous sentences (“Peter sees Susan with the telescope” - who has the telescope?) or sentences in which one word can have several meanings (“The astronomer marries a star” - what does star mean?). Incidentally, the common translation systems still mistranslate the sentence “The astronomer marries a star” - try it out!”
Artificial neural networks: how do you use them in your research field?
Prof. Tkatchenko: “What we really do is building tools. At the moment, we are making foundation models (like ChatGPT) of biomolecular dynamics. Basically, we are developing a specialized model trained on all the data that we and others have produced over the last 20 years on quantum mechanical calculations of molecular fragments. We demonstrate that once we train such a model, we can apply it to simulate biomolecular systems that are not there in the data. This means that we can assess a much larger range of questions. All biomolecules are dictated by quantum mechanical interactions; so, once you have a model that has learned those interactions from the data, in principle, eventually you could even simulate a whole cell. This really pushes the field.
In quantum chemistry, ML produces a lot of useful data. For example, you can calculate the properties of drugs (like bioavailability) or of materials like solar cells. ML changes the way that we ask scientific questions: what if I want a molecule or a material that has all the properties that I need, instead of computing the properties of a set of molecules?”
Prof. Schommer: “We use ML in countless areas. The exciting thing about this technology is its versatility: because it is a problem-solving tool, it can be used in almost any field. We are working on chatbots to stimulate communication between people, on the (semi-)automatic summarization of texts, on topics in the field of artificial creativity (what distinguishes a deep fake from a master painting?); even in subjects such as ethics, law or history, we deal with issues relating to the impact of machine learning on society. For example, we are currently developing a learning system to explain the First World War in collaboration with the C2DH."
In your opinion: What are the opportunities and risks of machine learning models?
Prof. Tkatchenko: “To me, AI is more intelligent than us in many domains, but much more ignorant in others. The danger comes from not understanding the tools we use. If we use an algorithm without understanding how it works, and this leads to new results, is this really a breakthrough, or a hallucination? With Chat GPT, those hallucinations are easy to debunk but in molecular simulations, things are much more complicated. That could be a danger.
On the other hand, we still don’t understand in all glorious details why planes fly (and when they fail), but we still use them daily. So, it’s perfectly fine to use ML, but we have to decompose it little by little to understand all the pieces. One needs new standards for new science, and ML is a new science.”
Prof. Schommer: “There are indeed certain drawbacks, such as the explainability of results or the use of programming libraries without fully understanding the architectures they contain. This also leads to people accepting results and relying on them, but not having the necessary basic knowledge to question them.”
Author: Diane Bertel
Editor: Michèle Weber (FNR)