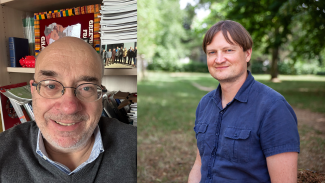
Der heutige Nobelpreis für Physik wurde an John Hopfield and Geoffrey Hinton für ihre „grundlegenden Entdeckungen und Erfindungen“ im Bereich maschinelles Lernen vergeben. Maschinelles Lernen (ML) ist ein Teilbereich der Künstlichen Intelligenz (KI), in der Computer eingesetzt werden, um aus Daten zu lernen und Muster zu erkennen. ML-Algorithmen benutzen mathematische Modelle, um Vorhersagen oder Entscheidungen zu treffen.
Wir haben mit zwei Experten von der Universität Luxemburg über den Nobelpreis gesprochen: Prof. Christoph Schommer, Associate Professor in Artificial Intelligence, und Prof. Alexandre Tkatchenko, Full professor in Theoretical Condensed Matter Physics. Beide nutzen Machine Learning in ihrer Forschungsarbeit.
Können Sie in ein paar Sätzen den heutigen Nobelpreis erklären? Inwiefern ist er im Bereich Machine Learning und KI-Forschung gerechtfertigt?
Prof. Schommer: „Der Nobelpreis ist an zwei Wissenschaftler vergeben worden, die beeindruckende Pionier-Arbeit geleistet haben. Erste Anfänge finden sich bereits in den 60er Jahren. Die Grundidee war, mit Hilfe mathematischer Modelle die neuronalen Aktivitäten zu simulieren, sprich, die Funktionsweise des menschlichen Gehirns nachzuahmen. ML ist nicht nur ein mathematisches Modell, sondern eine interdisziplinäre Disziplin, die der Bionik naheliegt: die Natur wird als Vorbild genommen. Im Laufe der Zeit ist es zu einer fantastischen Weiterentwicklung gekommen.“
Prof. Tkatchenko: “Machine Learning is a set of methods which is trained on data. It is trying to make a representation of the problem you are interested in, given data. That is very different from the traditional physical approach, where you first have a model with a fixed representation that you can then adjust with the data. Here, you do it the other way round. That change in perspective is quite revolutionary.
The first artificial neural networks that have been developed, the Hopfield networks (Ed. note: named after the current Nobel Prize winner, see infobox), came from statistical physics; they were the first generation. Then the algorithms were trained and made more powerful, and now they are used in all domains of science and technology. For that reason, for that fundamental, foundational development, the Nobel Prize is very justified.”
Welchen Einfluss hatte ML auf Ihren eigenen Werdegang?
Prof. Schommer: „Die 80er Jahre wurden durch die Weiterentwickelung der KI im Allgemeinen und auch der neuronalen Netzwerke im Speziellen geprägt. Damals habe ich die Hopfield Netzwerke (NDLR: benannt nach dem heutigen Nobelpreisträger, siehe Infobox), andere Neuronale Netz-architekturen und auch den Konnektionismus studiert und war sofort fasziniert von der Frage, ob und wenn ja, wie wir künstlich das kognitiv-bedingte Problemlösen simulieren können. In einer meiner Arbeiten beschäftigte ich mich der Disambiguierung von mehrdeutiger Sätzen, also etwa syntaktisch mehrdeutigen Sätzen ("Peter sieht Susan mit dem Fernrohr" - wer hat das Fernrohr?) oder Sätzen, in denen ein Wort mehrere Bedeutungen haben kann ("The astronomer marries a star" was bedeutet „star“?). Im Übrigen übersetzen die gängigen Übersezungssystem den Satz "The astronomer marries a star" immer noch falsch - probieren Sie es doch mal aus!“
Prof. Tkatchenko: “My bachelor’s degree was in computer science, but neural networks at the time were not very impressive. They were already very interesting though as a tool for developers. You could use neural networks to compress songs in WAV format; that was before we had MP3. You lost some accuracy, but the song was recognisable.
10 years later, ML was a completely different field. It was not so much a single breakthrough, rather than multiple ones in many directions that revolutionised ML: computer hardware, software, algorithms… This is why NVIDIA is now a 3 trillion Dollar company! The computer architecture is becoming more and more powerful, and that makes our progress in quantum chemistry and physics possible.”
Welche Forschung betreiben Sie und Ihr Team im Bereich Machine Learning?
Prof. Schommer: „Wir benutzen ML in unzähligen Gebieten. Das spannende an dieser Technologie ist ihre Vielseitigkeit: da sie ein Werkzeug zur Problemlösung darstellt, kann man sie in nahezu jedem Bereich verwenden. Wir arbeiten an Chatbots, die die Kommunikation zwischen Menschen stimulieren sollen, an der (semi-)automatischen Zusammenfassung von Texten, an Themen aus dem Bereich der Künstlichen Kreativität (was unterscheidet ein Deep Fake von einem Meistergemälde?); selbst in Fächern wie Ethik, Jura oder Historie arbeiten wir an Themen, die die Auswirkungen von Machinellem Lernen für die Gesellschaft betreffen. Aktuell entwickeln wir z.B. auch ein Lernsystem, um den ersten Weltkrieg zu erklären, in Zusammenarbeit mit dem C2DH."
Prof. Tkatchenko: “What we really do is building tools. At the moment, we are making foundation models (like ChatGPT) of biomolecular dynamics. Basically, we are developing a specialized model trained on all the data that we and others have produced over the last 20 years on quantum mechanical calculations of molecular fragments. We demonstrate that once we train such a model, we can apply it to simulate biomolecular systems that are not there in the data. This means that we can assess a much larger range of questions. All biomolecules are dictated by quantum mechanical interactions; so, once you have a model that has learned those interactions from the data, in principle, eventually you could even simulate a whole cell. This really pushes the field.
In quantum chemistry, ML produces a lot of useful data. For example, you can calculate the properties of drugs (like bioavailability) or of materials like solar cells. ML changes the way that we ask scientific questions: what if I want a molecule or a material that has all the properties that I need, instead of computing the properties of a set of molecules?”
Welche Risiken birgt diese Technologie?
Prof. Schommer: "Es gibt in der Tat gewisse Nachteile, etwa die Erklärbarkeit von Ergebnissen oder die Anwendung von Programmierbibliotheken, ohne die darin enthaltenen Architekturen komplett zu verstehen. Das führt eben auch dazu, dass man Ergebnisse annimmt und sich darauf verlässt, jedoch nicht die nötigen Grundkenntnisse besitzt, sie in Frage zu stellen."
Prof. Tkatchenko: “To me, AI is more intelligent than us in many domains, but much more ignorant in others. The danger comes from not understanding the tools we use. If we use an algorithm without understanding how it works, and this leads to new results, is this really a breakthrough, or a hallucination? With Chat GPT, those hallucinations are easy to debunk but in molecular simulations, things are much more complicated. That could be a danger.
On the other hand, we still don’t understand in all glorious details why planes fly (and when they fail), but we still use them daily. So, it’s perfectly fine to use ML, but we have to decompose it little by little to understand all the pieces. One needs new standards for new science, and ML is a new science.”
Autor: Diane Bertel
Editor: Michèle Weber (FNR)