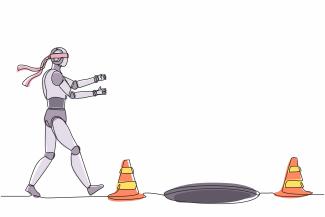
AdobeStock/Simple Line
AI tools cannot see what surrounds them – it’s as if they are blindfolded. Their best hope is to expect their target data to resemble that from their training period. Which is not always the case.
This article won the third place in the 2024 Science Writing Competition run by the University of Luxembourg in collaboration with science.lu.
From the moment we wake up, we make the same mistake of thinking that everything that was yesterday, will still be the same today. That the sun will rise in the east as it did before, or that the water that quenched our thirst will not poison us today. And we do so quite sure of ourselves because it worked up until now. But things do change. From one day to the next, people who greeted us with two kisses may now wear a mask and refrain from getting into contact. What once was cool may be outdated now.
No matter how many observations we make, we cannot be certain that future observations will follow the same pattern as they did in the past. Likewise, conventional artificial intelligence (AI) tools are trained on a limited set of examples and can only provide answers from the predefined set of answers they were trained on. If a prompt does not fit any known data, the AI tool will still produce an answer from what it learned, leading to mistakes. For instance, when COVID first appeared, an AI tool would have diagnosed something else, like tuberculosis or pneumonia, because the AI tool hadn’t yet learned about the new disease now called COVID.
Fighting blindfolded
This is not new. In ancient Rome, there were gladiators called Andabatas who used to fight blindfolded in the arena, unaware of the beasts waiting for them outside. Regardless of their training, they were doomed to fail. Similarly, we are told AI tools are black boxes whose complex insides cannot be inspected, but equally dangerous is the fact that AI tools cannot see what surrounds them. Like our gladiator, their best hope is to expect their target data to resemble that from their training period.
But AI is no exception to this. Scientists struggle with this problem in their daily work too. Let’s consider our omelet problem again and dissect our reasoning. It goes as follows: The first two eggs in the box were good. All the eggs expire on the same date. Therefore, we assume that the next egg will be good too. Sounds reasonable, but it is easy to imagine a situation where the next egg is rotten. We use this type of reasoning all the time. We take our phone, and we don’t expect it will explode when we unlock it. Although unlikely, we can imagine the possibility of it blowing up. This method of reasoning is known as inductive reasoning and scientists use it all the time too.
From the specific to the general
Scientists conduct their experiments a set number of times and then stop, convinced that the next run will yield the same result. Just like we did with our omelet. But 280 years ago, a philosopher came up with a problem that is still unsolved: David Hume said that when we reason inductively, we assume that nature is uniform. If we throw a stick up in the air, we expect it will always fall. And here comes the punch line. He says that the only way to justify our belief in the uniformity of nature is with induction itself, which leads us to a vicious circle.
Hume was a radical, and concluded that induction cannot be rationally justified at all even if we use it every day with great success, because we can always imagine a situation where nature behaves differently.
Recovering our faith in science
According to Hume, scientists can never be entirely sure of their results. As Albert Einstein said: “No amount of experimentation can ever prove me right; a single experiment can prove me wrong.”
This realization may leave an empty feeling that science is indeed fallible, but it is this very gap that scientists work hard to fill. We can imagine the building we live in collapsing or the train that takes us home derailing, but architects and engineers built them with a factor of safety. Likewise, scientists seek robust confidence intervals, so intervals that theoretically contain the true value of the parameter they measure or estimate. This influences how they design their experiments, from the size of their studies to the methods they use.
Removing the blindfold from AI
Not everything is lost for our AI gladiator. Scientists at Luxembourg’s research institutions continue to investigate new ways for AI tools to identify when an input does not belong to any of the known categories and then either reject it or flag it for later human inspection. Also, students from the Master of Data Science at the University of Luxembourg learn about the risks of AI and how the scientific method can help to overcome them. Moreover, the Luxembourg Institute of Health explores the challenges of AI for the future of healthcare.
The latest AI solutions like generative AI can produce more diverse outputs, but they still depend heavily on the training data. However, just as we humans continuously learn from the world, strategies like continual learning, which means learning from continuously changing datasets, aim to constantly update AI tools to rapidly adapt to new scenarios. In the end, induction remains a useful tool for our daily lives, and with the proper methods, it will be useful for AI too.
Author: Carlos Vega (Luxembourg Institute of Health)
Editor: Michèle Weber (FNR)
Infobox
From the author:
From Hume to Wuhan: An Epistemological Journey on the Problem of Induction in COVID-19 Machine Learning Models and its Impact Upon Medical Research
https://ieeexplore.ieee.org/document/9475449
Analysis: Flawed Datasets of Monkeypox Skin Images
https://link.springer.com/article/10.1007/s10916-023-01928-1
Course class book: Philosophy of Science and Data Ethics
https://carlosvega.github.io/PoS-DE
From the general literature:
Bergadano, Francesco. 1991. “The Problem of Induction and Machine Learning.”
Ladyman, James. 2012. Understanding Philosophy of Science.
Okasha, Samir. 2016. Philosophy of Science: Very Short Introduction.
Pearl, Judea, and Dana Mackenzie. 2018. The Book of Why: The New Science of Cause and Effect.
Russell, Bertrand. 1912. The Problems of Philosophy.
Domingos, Pedro. 2012. “A Few Useful Things to Know about Machine Learning.”