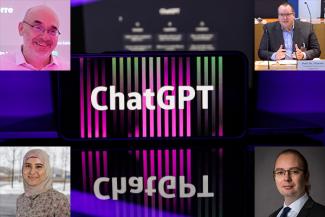
shotshop.com, uni.lu, Deutscher Bundestag, A.Tantar
Die Experten: Prof. Christoph Schommer (l.o.), Dr. Sallam Abualhaija (l.u.), Prof. Patrick Glauner (r.o.), Dr. Alexandru-Adrian Tantar (r.u.)
Seitdem die Forscher der Firma OpenAI ihr Chatbot ChatGPT für registrierte Nutzer öffentlich zugänglich gemacht hat, wird viel über darüber in den Medien diskutiert. Für die, die es noch nicht mitbekommen haben: ChatGPT ist ein Sprachmodell, eine künstliche Intelligenz (KI), mit der man sich nicht nur unterhalten kann, sondern die auch erstaunlich realistische Inhalte verfasst. Im Prinzip ist ChatGPT ein Textgenerator: man gibt eine Frage ein und erhält eine Antwort. Was unter anderem für die aktuelle Faszination sorgt, ist die hohe Qualität der Antworten, die ChatGPT liefert.
Doch inwiefern stellt ChatGPT in der KI-Forschung gerade ein Durchbruch dar? Was sind Chancen und Risiken solcher Sprachmodelle? Welchen Impakt haben solche Tools auf die zukünftige Arbeitswelt? Wie werden solche Sprachmodelle auch in Zukunft besser? Und welche Qualitäten haben wir Menschen, die nicht durch eine KI ersetzt werden können? Diese Fragen haben wir vier Forschenden aus dem Bereich der KI gestellt:
Hier die Antworten der Forschenden (auf Deutsch und Englisch):
Was denken Sie: Inwiefern stellt ChatGPT in der KI-Forschung gerade einen Durchbruch dar?
Prof. Christoph Schommer: Ich denke, es ist verfrüht, von einem Durchbruch zu sprechen. Schließlich liegen meines Wissens noch keine soliden und objektiven Erfahrungen bei der Nutzung von ChatGPT vor und solide Ergebnisse und substanzielles Feedback in der Anwendung sind gerade erst am Entstehen. Auch ist die Architektur von ChatGPT nicht wirklich bekannt: wie wird die Extraktion der Daten realisiert? Wird deep learning angewendet? Wie wird Sprache generiert? Es stellt sich auch die Frage, ob man von einem Durchbruch sprechen kann, wenn ChatGPT nachweislich Falsches wiedergibt bzw. auch nicht preisgibt, auf welche Quellen die Texte sich denn beziehen? Sicher nicht. Und: ab welchem Punkt kann man überhaupt von einem Durchbruch sprechen? Schließlich handelt es sich hier weder um ein mathematisches Theorem noch um eine physikalische Behauptung. Das allgemeine Interesse an der datengesteuerten KI und dem damit verbundene Hype lassen chatGPT durchaus als etwas ganz Besonderes erscheinen. Aber im Moment sehe ich ChatGPT erst einmal nur als einen vielversprechenden, und nützlichen Ansatz, der die Welt bereits verändert hat und dies auch weiterhin tun wird. Ich bin mir sicher, dass andere Global Players folgen werden; und so ist ChatGPT ein Startschuss für zu erwartende Investitionen. Wahrscheinlich wird es bei den Internetgiganten zu Marktverschiebungen kommen; ein Wettlauf ist zu erwarten, denn: warum soll ich jetzt noch googeln, wenn ChatGPT mir gleichzeitig das Ergebnis erklärt? Aber selbst wenn sich diese Systeme durchsetzen, bleibt immer ein Restrisiko durch noch nicht ausdiskutierte Folgen ihrer Nutzung.
Prof. Patrick Glauner: Von der Anwendung her ist es ein Durchbruch, aber nicht von der Methodik. Es gab ja schon das Sprachmodell GPT-3, das vor ein paar Jahren auch etwas Aufmerksamkeit in den Medien bekam, dann wieder weniger. ChatGPT ist im Wesentlichen eine Weiterentwicklung von GPT-3: es wurden vor allem Verfahren verbunden die es schon gab.
Dr. Sallam Abualhaija: ChatGPT represents an exciting development in chatbot technology, as it has been developed with human input, making it seem more natural. It is based on GPT-3, a large-scale language model (LLM)*1 that has extensive language capabilities because it is pretrained on large text bodies. Most large-scale LLMs are trained on text without direct feedback from humans describing their preferences. For example, LLMs can generate multiple responses to the same question, but not all of them are equally good from a human’s perspective. Aligning language models with the human preferences user's intention is however crucial to advance language technologies to interact with humans in tasks like auto-completion for texts or dialogue.
ChatGPT has set its sights on this goal and aims to provide responses to conversations that are both meaningful (from a language-perspective) and useful (from a user-perspective). Current research is focused on developing even more powerful AI-based systems which would in turn better interact with and assist humans.
In view of the rapid progress in the landscape of natural language processing (NLP)*2 technologies over the past decade, I believe that ChatGPT is indeed a prominent achievement in the field. However, I think this is only the beginning. The chatbot is currently verbose and repetitive. The next iteration of the language model it uses, GPT-4, will have improvements based on the experiences with humans that ChatGPT is currently collecting. It will be even more capable of meeting the higher expectations that humans have about AI.
*1: Large-scale language models (LMs) are trained on / exposed to large bodies of text to predict the next word given preceding words within some text. During training, the models learn contextual information, regularities of language, and syntactic and semantic relations between words.
*2: Natural language processing (NLP) is a sub-field of AI that explores how computers can analyze, attempt to understand, and generate natural language (text and speech).
Dr. Alexandru-Adrian Tantar: ChatGPT builds on a model referred to as GPT3.5 which, in itself, is intended as a prequel for GPT4, expected to be released by OpenAI in 2023. The precursor of ChatGPT, GPT3, was and still is, to a certain extent, a more powerful model, with a more general-purpose reach. ChatGPT, to this end, is not unique nor, from that general-purpose standpoint, the most advanced. OpenAI, moreover, provides not one but a number of other models in this same GPT3 family, e.g. text-davinci-003, text-curie-001, etc., less known to the general public, overall offering different trade-offs between the complexity of the tasks they can perform and speed. All of this, ultimately, bares, of course, the question of ‘What makes ChatGPT different? Does it stand for a breakthrough?’
GPT3 was a breakthrough while in itself, it was not ‘impressive’ in terms that may relate to or be appealing to the wider public, not on the same scale as what we’re now seeing with ChatGPT. ChatGPT is, at the same time, trained specifically for complex conversational tasks, standing as the most advanced conversational model to date and, in some sense, a breakthrough if looking at the level of interaction it supports. In this sense, ChatGPT continues a line of Large Language Models (LLMs), with billions of parameters, and where the sheer amount of input, quality data will, in the future, likely play the more important role.
Closing on the question and anticipating what may come next, Sam Altman, the CEO of OpenAI, commented on GPT4, i.e. the next model OpenAI will release, stating that “People are begging to be disappointed and they will be. The hype is just like... We don’t have an actual AGI [Artificial General Intelligence - a machine that can perform any intellectual task that a human can, (note of the editors)] and that’s sort of what’s expected of us.” In the meantime, Google announced Bard, Baidu steps in with ERNIE, and other models and/or applications will surface. What is most interesting, the wide-spread use and new applications such models will support, is still to follow – an AI race.
Was denken Sie: Was sind Chancen und Risiken solcher Sprachmodelle?
Prof. Christoph Schommer: ChatGPT bietet viele Chancen in seiner Anwendung und birgt das Potenzial, eine Komponente für kreative und komplexe Prozesse zu sein. Persönlich denke ich, dass ChatGPT & Co. zum persönlichen Lernbegleiter und damit zu einem individuellen Assistenten wird. Auch ist eine Integration in einen Roboter denkbar. Eine Integration im Arbeits- und Freizeitbereich wird stattfinden und das Leben (zunächst) erleichtern. Ich sehe ChatGPT grundsächlich als Chance für den Bildungserwerb, was allerdings Konsequenzen im Wissenserwerb, im Vermitteln von Wissen und in deren Überprüfung haben wird. Unter Umständen wird es zu Konflikten kommen, denn: wenn ChatGPT und die Lehrer unterschiedlicher Meinung sind, wem soll der Schüler denn dann glauben? Und: wird man chatGPT, der digitale, aber verlässliche, künstliche Kompanion, der auf alles eine Antwort hat, einfach so ignorieren können? Ein Risiko liegt darin, ChatGPT’s Ansichten kritiklos zu akzeptieren und widerstandslos zu übernehmen, etwa aus Bequemlichkeit. Denn natürlich lockt das ChatGPT-Versprechen, schnell und unkonventionell zu helfen, auf der anderen Seite aber droht eine Abhängigkeit, ohne die es dann auch nicht mehr geht. Andere Risiken sind: wenn in das Sprachmodell Daten, deren Ursprung nicht wirklich klar ist, eingespeist werden, können wir denn dann sicher sein, dass das Resultat denn auch wirklich stimmt? Haben die Ergebnisse Tiefgang oder ist es nur Effekthascherei? Können wir die Kausalitäten nachvollziehen und verstehen, was das Ergebnis wirklich bedeutet oder ist es ein: „egal, das wird schon stimmen“? Aus technischer Sicht gestalten sich Fragen wie zum Beispiel: wie lange braucht es, um aktuelle Ereignisse in das Large Language Model mit einzubeziehen bzw. zu aktualisieren? Ist es in Ordnung, einen Text oder einen Softwarecode als einen Prozess eigener Kreativität zu betrachten? Wie beweise ich ein Plagiat?
Prof. Patrick Glauner: In punkto Chancen bietet ChatGPT eine Möglichkeit, viel schneller und günstiger bessere Texte zu generieren. Es bietet also eine Effizienzsteigerung für die Menschen. Was die Risiken betrifft, so werden solche Sprachmodelle natürlich Veränderungen auf dem Arbeitsmarkt mit sich bringen. Außerdem kann so ein Sprachmodell natürlich totalen Unsinn extrem überzeugend darstellen, was bedeutet, dass alle Inhalte überprüft werden müssen und man dem Programm nicht blind vertrauen kann.
Dr. Sallam Abualhaija: Large-scale language models (LLMs) like the one ChatGPT is based on (GPT-3) have already demonstrated remarkable performance. Such models develop their extensive knowledge of language by being exposed to a huge amount of text. With that they enable innovative, efficient, and accurate solutions to be built, e.g., improving search engines with question answering features. I believe that LLMs can effectively collaborate with humans in diverse contexts where understanding and/or generating new text is required.
However, this extensive knowledge might not always be adequate for devising accurate automated solutions in specific industries, such as the legal or finance sector, where the problems are highly complex.
LLMs, and AI technologies in general, have introduced new challenges concerning ethical principles. For instance, how would ChatGPT converse with a human being asking about robbing a bank? Does ChatGPT realise the consequences of providing "accurate" answers? LLMs, including GPT-3, are pre-trained on text from the web, they are thus naturally prone to different facets of bias, generate toxic text, and can easily make factual errors due to the outdated knowledge they learned.
Another concern is related to sustainability. The larger the models are, the more computing resources (i.e., energy) are needed for their training. Compared to previous generations of advanced language technologies, each new generation is taking about 10 times as much energy as the last model. This is growing by orders of magnitude, to achieve linear improvement in performance. With the advent of such a breakthrough natural language processing (NLP) tool, which has captured the attention and is being used by the general public, we need to remain aware of the consumption of computational resources that supportsevery generated text, and the ethics behind the model.
Just like other AI technologies, LLMs should manifest being worthy of trust and valuable to be adopted in practice. AI technologies should be regulated; more transparency and ability to audit are needed.
Dr. Alexandru-Adrian Tantar: The main risk is with us, at a more human and societal level, from the use we make of such models.
First, from looking at models like ChatGPT for more than what they are: mathematical functions, albeit complex and building on different mechanisms, fine-tuned to capture, in a simplified way of seeing things, correlations over the input data. Think of ‘fruits and …,’ or ‘up or …’ A plausible completion would be ‘vegetables’ and ‘down,’ respectively. Models like ChatGPT function by tuning parameters to capture such relations in the data while, true, at a different level. Writing poetry in the style of Shakespeare, answering questions on Quantum Computing, or generating Python code, is of course not as simple as matching words. The principle, however, stands, in some sense, the same.
Where’s the risk? Us looking at such models as if being in front of a mirror and not being aware of the number crunching machine behind. Not being critical. Models like ChatGPT reflect mechanically, though true, in a smart way, our knowledge, our way of building an answer and based on a reasoning that the input does not expose in an explicit manner. Moreover, it is us reading and reasoning about the answers. But does the AI ‘understand’ what it ‘says?’ Not to mention that, while the model may sound convincing in what it outputs, it builds on our own knowledge which, to start with, may be biased, not fully aligned on ethics or, worse, incomplete.
Second, and linked to the above, a separate risk lies with how the model training works. Such models ‘average’ over data. What is the answer for ‘Is the glass half full or half empty?’ How about more complex questions or that may be borderline w.r.t. societal implications? Q&A models, when data intrinsically induces confusion, finally blur the answer and pick what was seen the most in the training (which does not equate ‘right’), or some interpolation between possible or, at times, conflicting answers. ChatGPT may confidently provide an answer for ‘How much is pi/e?’ but will it be based on reasoning and maths or simply examples it has seen ‘out there’ with the training data? We basically need to go back to questions like ‘How do we build trust, how do we embed mechanisms for e.g. knowledge representation and reasoning verification?’
Was denken Sie: Welchen Impakt haben solche Tools auf die zukünftige Arbeitswelt?
Prof. Christoph Schommer: Ich denke, dass die Menschen den Weg des geringsten Widerstands gehen bzw. den Weg, der Ihnen am meisten nützt. Ähnlich wie bei den verfügbaren digitalen Übersetzungstools wird chatGPT seinen Weg in die Arbeitswelt finden. Ich kann mir vorstellen, dass Standardschreiben wie Briefe, Einleitungen/Übersichte zu einem Thema, Literaturrecherche, einfache Programmierlösungen, aber auch kreatives Arbeiten verstärkt durch oder in Zusammenarbeit mit ChatGPT stattfinden wird. Zumindest im letzten Fall wäre eine Transparenz und ein Label à la „Made by ChatGPT“ notwendig. Ich fürchte, dass der Grad der Nützlichkeit den eigenen, zu erbringenden, Aufwand übertrumpfen wird. Der Begriff ‚Leistung‘ wird sich anders definieren müssen. Meine Vermutung ist: Wenn ChatGPT so erfolgreich ist und Einzug ins Berufsleben erhält, dann wird eine gegebene Aufgabe in weniger Zeit realisierbar. Das würde bedeuten, dass man mehr Aufgaben vergeben kann. Möglicherweise wird also die tägliche Arbeit erleichert, sich wohl aber erhöhen. Was den Bereich der Ausbildung anbelangt, so werden Schüler und das Lehrpersonal ChatGPT für unterschiedliche Zwecke und in unterschiedlicher Intensität anwenden. Der Lehrer wird zum Architekten der Wissensvermittlung und ChatGPT ein persönlicher Lernbegleiter für alle. Eine Überprüfung der zu erbringenden Leistungen kann somit nicht mehr ausschließlich schriftlich, sondern muss verstärkt auch mündlich (und in eigenen Worten) erfolgen. Auch wird das Lehrpersonal zunehmend mit den von ChatGPT gegebenen Antworten, Inhalten, Meinungen, Ansichten konfrontiert werden: denn ChatGPT ist nun der Experte, der digitale Professor, der auf alles eine Antwort hat. In Disziplinen wie in der Gesundheitsfürsorge, der Rechtsprechung, in gesellschaftspolitischen und religiös-bedingten Angelegenheiten ist äußerte Vorsicht geboten. Denn solche Systeme sind immer auch ein kommerzielles Produkt, hinter dem jemand steckt. Interessen, Meinungsbildungen und damit Einflussnahmen sind deswegen nicht auszuschließen. Aus Sicht der Wissenschaft hingegen gilt nach wie vor das Gesetz der Neugier und der Schaffenskraft. Ein Zusammenspiel und Diskussion aller Parteien scheint notwendig.
Prof. Patrick Glauner: Das ist der Anfang eines großen Wandels. Es wird große Veränderungen im Arbeitsmarkt geben und das nicht nur im Bereich der Erzeugung von Texten, sondern auch Bildern, Videos, und Audio. Weil man mit solchen Tools viel schneller und günstiger gute Inhalte generieren kann wird das natürlich besonders Agenturen und Content-Ersteller treffen, die solche Inhalte bisher produziert haben. Es werden also auch einige Jobs im Laufe der Zeit verschwinden. Es werden aber auch neue Jobs entstehen, z.B. werden in Zukunft dann mehr KI-Experten benötigt, die solche Modelle weiter entwickeln können, oder sogenannte AI-Prompter, die einer Künstlichen Intelligenz Anweisungen gibt.
Zudem bin ich der festen Überzeugung, dass durch diesen Wandel auch unser Wohlstand gesteigert wird, und der Mensch bekommt mehr Zeit für andere Dinge, die ebenfalls wichtig sind.
Dr. Sallam Abualhaija: Emerging AI technologies introduce opportunities and challenges at the same time. I believe that AI will empower humans in their work activities, where AI takes over some load of repetitive tasks (e.g., extracting and categorizing information from legal documents) and leaves humans with ample time for more critical tasks (e.g., making decisions about liability).
The discussion about humans being replaced in their jobs is something that reoccurs, with every new technology that makes noise on the market. Looking back in time, when old jobs vanished, new ones emerged. The majority of jobs remained in practice, and probably will, but we will see reliance on intelligent automation increase in those roles.
For instance, incorporating LLMs in businesses for the purpose of translation has a significant impact on improving sales, opening new markets, but with more sales comes more customers that need humans to support them with their purchases, keeping the value of the human with the linguistic skill set.
Dr. Alexandru-Adrian Tantar: The immediate and obvious impact is in the way we search for and use information. No surprise that Microsoft, Google, etc., compete in exactly this area, today. And this goes further than simply retrieving text or data from the Internet using a search engine. Think of models that step beyond being conversational and that are true assistants with automating tasks in the ‘white collar’ world. Recommending on strategic business decisions, for example, while being connected with the most up to date information. Lots of information. The medical domain, law, computer science, finance and regulation, etc., all are likely to experience changes over the next years. And not the mundane, simple aspects. What happens if we combine models like ChatGPT, and future versions of it, with the metaverse? (a virtual world where people can interact and experience a shared digital space; note from the editors) How about avatars, presence on social media, or personal assistants, extended presence, how about personalised education, accompanying the elderly, etc.? How about a judge ruling based on input from ChatGPT? It already happened, in Colombia, questionable as it may be from an ethical point of view. Such applications are already being explored… it’s just that we get back to that point and question of building trust. Do we trust the AI? At some moment in the future, it will be ‘Do we trust the Human?’ But now, now, do we trust the AI? We may confidently drive around using the path an AI suggests. But how about a complex diagnostic, legal case, etc.? Then, what’s important to retain is ‘automate.’ First, second, third, …, wave and short-term evolutions of such models, will for all areas of application still function with the human counterpart in a position of validating and, more importantly, taking responsibility for what is retained as output. At the very end, however, such models will in the longer term, remove the repetitive or cumbersome part of our work, in the same way that the steam engine, electricity, or computers did. Then, over the short term, we’d still need to observe filters from the regulatory area – see EU’s AI Act and upcoming AI regulatory framework (equivalent of the GDPR but for AI), or as driven by societal push-backs/acceptance.
Was denken Sie: Wie werden Sprachmodelle auch in Zukunft besser? Durch mehr Rechenkraft und Skalieren von Trainingsansätzen? Oder sind auch andere Ansätze nötig?
Prof. Christoph Schommer: Mehr Daten und mehr Rechenkraft bedeuten nicht automatisch auch ein besseres Modell. Stattdessen halte ich eine Qualitätsüberprüfung der Daten für notwendig sowie auch die Qualitätsüberprüfung der generierten Antworten. Diese müssen auf verlässlichen Quellen beruhen, auf überprüften Fakten, und weder subjektiv sein noch diskriminierend. Die Antworten sollten dem Alter des Nutzers angepasst sein, denn ein Jugendlicher versteht Zusammenhänge anders als ein Erwachsener. Verbesserungen erwarte ich in der Interaktion mit dem Benutzer, so interagieren wir zurzeit noch mit einer schriftlichen Eingabe, warum denn nicht auch mündlich? Wird Englisch nach wie vor die wichtigste Sprache bleiben oder ist eine direkte Wissensverarbeitung, etwa mit Quellen in Luxemburgisch, Persisch oder Japanisch (ohne den Umweg: Englisch) auch bald denkbar? Wird ChatGPT bald mein persönlicher Assistent sein, mein Stellvertreter, etwa in direkter Konversation mit einem anderen ChatGPT?
Prof. Patrick Glauner: Der Ansatz, der bisher genutzt wurde ist das Deep Learning, also das Trainieren der sehr großen KI-Modellen anhand von großen Mengen an Texten. Diese Methode stößt aber langsam an ihre Grenzen und es werden neue Ansätze nötig sein. Die aktuellen Sprachmodelle sind nicht wirklich intelligent: sie bringen nur Wörter miteinander in Verbindung. Um die Sprachmodelle so weiter zu entwickeln, dass sie nicht nur Korrelationen sondern auch Kausalitäten verstehen – also nicht nur Muster in Daten erkennen, die sich ähnlich verhalten, sondern das Verhältnis zwischen Ursache und Effekt bestimmen.
Außerdem sind die aktuellen Trainingsmethoden nicht nachhaltig, da sie sehr viel Strom benötigen. Hier besteht also Forschungsbedarf.
Dr. Sallam Abualhaija: Larger is not always better. The success behind ChatGPT is based on better interaction with humans.
Building larger models like GPT-3 has nonetheless shown promising results in obtaining human-like behavior by learning from only a few examples of natural language processing (NLP) tasks. All language models are originally trained within a context to predict the next word. Despite the simplicity of this objective, it is a very effective task that gives models wide understanding of semantics and structures of languages. This understanding is then employed to solve specific tasks, e.g., answering questions. Large models still have limitations, including for example dealing with the 'too many' exceptions that can occur in the real world, acquiring common sense capabilities, etc.
In the future, we need computers that understand human language. Language models (LLMs) should improve to be able to work smoothly together with humans in a collaborative environment. We want LLMs to be trained to achieve higher performance but at the same time require less computation, which would lead to more energy efficiency. We need to devise effective methods to update the knowledge of LLMs.
Dr. Alexandru-Adrian Tantar: Computing power will with no doubt play an important role. We already witnessed a dramatic change when moving from CPUs to GPUs (Note from the editors: Central Processing Unit and Graphics Processing Unit, see below* for more information). What will it happen once we have a business-viable Quantum Computer? Is the sheer power sufficient? Are more advanced models the key? Such points are for sure part of the answer. The full extent of it, however, is more than likely to ultimately depend on data. How much data can such a model be provided with, building trust, being able to correct for bias and incorporate real-time or recent information will be key (Google’s Bard is expected to build on the platform’s reach to what is world-wide continuously evolving data). The entity holding what may be reference data or providing mechanism to enforce trust, w.r.t. any business or societal sector, will be in a strategic position. Would you use an AI model if, to start with, you would not trust the data it has been trained on and if, downstream, no mechanisms to ensure what is now defined as AI trustworthiness are in place? What are the strategic risks, a small business may need to assume, in that case, or what is the impact for e.g. financial markets, AI-driven research, or national security? In the longer term, a different line of thought may be models that can derive knowledge that was not explicitly provided with/in the input data. Which, if attained, may finally end in an exponential of what such models can help us explore. What would it mean to ‘rediscover’ the laws of physics in a single day? How about what we don’t already know? Last, the combination of language models with reasoning, in more formal terms, is also a likely and immediate next step – for example a Large Language Model + Wolfram Alpha (a knowledge engine that provides answers to questions and computations based on a vast database of knowledge and algorithms; note from the editors). A key question in the next-steps evolution, and for all of those points, will be the AI-Human interaction. The AI being able to incorporate our values and us being able to understand the AI.
*A CPU (Central Processing Unit) is the component of a computer that does most of the processing, which means retrieving and executing instructions stored in memory and performing arithmetic and logical operations.
A GPU (Graphics Processing Unit) is a specialized type of processor optimized to perform many simple tasks simultaneously. This makes it efficient than a CPU for some types of tasks, for example the rendering of images and videos.
In simple terms, a CPU is like the "brain" of the computer, while a GPU is like its "muscles." The CPU manages and coordinates all the tasks and processes in the computer, while the GPU helps the computer perform these tasks faster and more efficiently, especially when it comes to processing of graphics and videos.
Welche Qualitäten haben wir Menschen die nicht durch KI ersetzt werden können? Sind das noch immer die 4K? (Kommunikation, Kollaboration, Kreativität und kritisches Denken?)
Prof. Christoph Schommer: Ich denke ja. Dazu gesellen sich aber auch noch andere kognitive Komponenten, wie etwa das Lernen, das Begreifen einer Kausalität, Abstraktionsvermögen, Adaptivität, Humor, Verständnis und der Entschluss zu Entscheidungen, die nicht immer einer Rationalität/Logik folgen.
Prof. Patrick Glauner: ChatGPT kann man schon eine gewisse Kreativität zuschreiben, daher würde ich sagen, dass die wichtigsten Qualitäten der Menschen weiterhin Kommunikation und kritisches Denken bleiben. Und wenn eine KI im medizinischen Bereich einem Arzt bei der Diagnose hilft, könnte das dem Arzt z.B. mehr Zeit für Kommunikation geben. Im Allgemeinen sehe ich künstliche Intelligenzen als unterstützend; sie sollen nicht alle Menschen ersetzen. Sie kann wohl auch Entscheidungen treffen, aber wenn es um Leben und Tod geht, soll eine KI nicht entscheiden.
Dr. Sallam Abualhaija: Even though AI is driving so many applications that we are interacting with in our everyday lives, I believe that humans will not be replaced by AI. Current AI is incomparable to human intelligence.
Dr. Alexandru-Adrian Tantar: A difficult question. And my personal standpoint – likely none. There is nothing that the AI will not be able to replicate. Which is not the same as replacing. Having creativity set as a target difficult to reach may be misleading and subject to a proper definition. Think of ‘simply’ noise in what are ‘normal’ patterns of our activities, and training models to either offer outputs that we, as humans, find interesting (poetry, paintings, music, etc.), or that, in the same sense, may be disturbing, for what art may stand for. Then, we do appreciate the life experience that may have led to a novel to be written and not just the text in itself. And this is something that the AI will lack. And we are however still far from the Artificial General Intelligence. With this, however, it should be straightforward that we passed some important milestones, and we may already observe an exponential of the AI that crosses our own line of evolution. An important aspect where it is difficult to anticipate what the next stage of AI will be, is digital consciousness. And not because of technical considerations; we are able to mimic highly complex cognitive processes, etc. It may be that we’ll end up mimicking, in the same way, consciousness. It is just that ‘What is consciousness?’ is the ‘hard problem.’ And, as we do not have a clear definition, we do not have the answer, which makes that it will likely be challenging to say that we have AI that replaces the human, consciousness included.
Autoren: Christoph Schommer, Patrick Glauner, Sallam Abualhaija, Alexandru-Adrian Tantar.
Redaktion: Michèle Weber, Lucie Zeches (FNR)
Fotos: Universität Luxemburg (Sallam Abualhaija), Deutscher Bundestag (Patrick Glauner), Alexandru-Adrian Tantar, Christoph Schommer
Infobox
Die Fragen an die Experten sind unter anderem angelehnt an folgendes Press Briefing des German Science Media Center: https://www.sciencemediacenter.de/alle-angebote/press-briefing/details/news/chatgpt-und-andere-sprachmodelle-zwischen-hype-und-kontroverse/