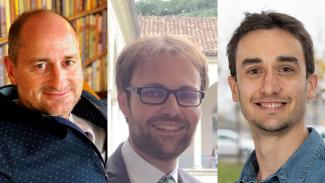
(C)Pierre Mangers/Christophe Ley/University of Luxembourg
Corona glossary authors: Daniele Proverbio (right), Christophe Ley (middle), Pierre Mangers (left)
Since the new corona virus entered our lives, we are increasingly confronted with technical terms that are otherwise only of importance to experts. All of a sudden, we have to deal with concepts such as reproduction number, virulence or the difference between PCR and antibody tests.
Daniele Proverbio, Christophe Ley and Pierre Mangers went through the trouble of creating an extensive glossary of terms related to the coronavirus pandemic. Daniele Proverbio is a PhD student at the Luxembourg Center for Systems Biomedicine (LCSB) at the University of Luxembourg; Christophe Ley is Professor of Mathematical Statistics at the University of Ghent and Vice President of the Luxembourg Statistics Association; and Pierre Mangers, a qualified chemical engineer and economist, heads his own consulting company MANGHINI Consulting after 20 years of professional experience in management consulting and industry. The three work together in Work Package 6 of the COVID-19 Task Force from Research Luxembourg, which deals with the statistical projections of the pandemic in the country.
For a better overview, we have structured the glossary as a drop-down menu. The terms are arranged alphabetically within the individual chapters. The complete glossary can be downloaded as a PDF at the end of the article.
science.lu would like to point out that the rapidity with which new information about SARS-CoV-2 and COVID-19 is released every day may make some of the information in this document out of date very soon.
Nonetheless, we hope that it provides a useful reference while also giving an insight into the complexity of the topic.
What follows is the text of the scientists:
1. Introduction
Effective communication in science relies on the understanding of specific terminology. This document provides a handy glossary of the words used to describe the Coronavirus disease-2019 (hereafter COVID-19 or pandemic), caused by the SARS-CoV-2 virus, and the work of researchers studying the disease.
The authors would like to underline the importance of scientific research in the current situation. Studying a novel virus requires interdisciplinary collaborations between many scientists in order to decode the nature of the virus, understand the pandemic and its impact on several sectors of our society. Each area of expertise plays a specific role. Universalists (scientists with a broad overview) assess the pandemic globally at the beginning to identify the big picture of the disease and the interwoven subject matter expertises required to get it under control. Specialists intervene to deliver their deep subject matter expertise. It is crucial to rely on both to communicate about different aspects of the pandemic. For this reason, the following glossary is covering a wide diversity of scientific fields, with words that relate to COVID-19 and health-realted topics but also to mathematics, modelling and research in general.
2. Glossary
CORONAVIRUS PANDEMIC
SCIENTIFIC RESEARCH
HEALTH-RELATED TOPICS
About the authors
Complete glossary for download as a PDF.
Authors: Daniele Proverbio, Christophe Ley, Pierre Mangers
Editors: Lucie Debroux (LCSB), Michèle Weber (FNR), scienceRELATIONS
Find the German and French version of the glossary here: